
In Figure 1 above, we see an output mask example for each method. Figure 1: The difference between semantic segmentation and instance segmentation ( source) Instance segmentation allows users to not only segment the region of interest from the original image but also to classify what that region of interest is (e.g. The main difference between these two is that in semantic segmentation a pixel-level classification is performed directly, while in instance segmentation approaches an additional object detection step is needed to obtain the individual instances of all classes in an image. Segmentation methods based on pixel-wise classification can be roughly divided into two categories: semantic segmentation and instance segmentation. Semantic Segmentation vs Instance Segmentation Known as pixel-wise binary classification, a mask with exactly the pixels that belong to each region of interest is inferred. The cell segmentation task localizes cell object boundaries in an image and distinguishes these object boundaries from the background of the image. This article, however, will not discuss other medical/structural segmentation. In the latter method, one might focus on whole-cell segmentation in the 2D microscopy images where the cytoplasm appears bright, the background is dark, and the nucleus has little or no staining. Oftentimes, the term cell segmentation has been used to refer to the segmentation of the cell nuclei (nuclear segmentation) as opposed to segmenting the entire cell body including the cytoplasm (nuclear + cytoplasmic segmentation). Let's dive in! How Cell Segmentation Works Scope of This Article In this article, we will walk through the following topics to give you an idea of what cell segmentation is and how you can start using it for your use case:
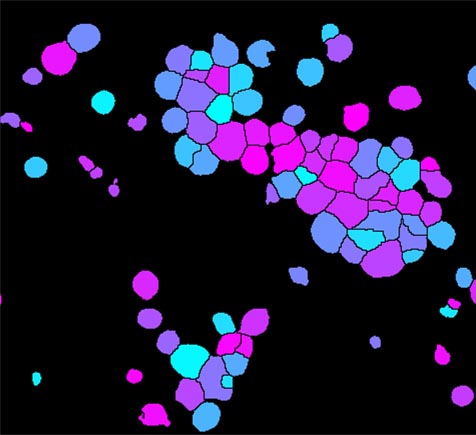
These insights can drive drug discovery, diagnostics, and other important fields of biology, pharmacology, and personalized medicine. Scientists can quickly evaluate how these features change over time and in response to a variety of conditions. Using cell segmentation, scientists are able to analyze relevant biological features such as cell count, type, division, shape, etc. Coupled with more compute power and new algorithms in machine learning and computer vision, cell segmentation has become a lot more efficient and accurate.Ĭell segmentation, or identifying cells in an image, has emerged in the past few years as a commonly used technique crucial for image-based cell biology research. Recently, breakthroughs in high-resolution fluorescent microscopy have enabled detailed visualization of cells and their subcellular components. Cellular dynamics that are characterized in normal and pathologic conditions continue to enhance our understanding of basic biological processes in addition to accelerating drug discovery.
#Cellprofiler separate cells by shape driver
The ability to image, extract, and study cells and their subcellular structures is crucial to several research areas and is a driver of advances in biology, medicine and drug discovery.
